©2021 Reporters Post24. All Rights Reserved.
Google most certainly raises eyebrows when it comes to AutoML. In May 2021, when the tech giant announced the general availability of Vertex AI – which brings AutoML
and AI platforms together into a unified API, client library and user interface – it changed the way enterprises look at deploying and maintaining AI models for the better.
The software requires nearly 80 per cent fewer lines of code to train a model versus competitive platforms. Thus, enabling data scientists and ML engineers across all levels of expertise to implement machine learning operations to efficiently build and manage AI/ML projects throughout the entire development lifecycle.
How To Start Your Career In Data Science?
Fast-tracking the AI/ML production in the wake of shifting market dynamics, Vertex AI brings together the Google Cloud services for building ML under one unified UI and API, simplifying the process to building, training, and deploying AI/ML models at scale. Thanks to decades of innovation and strategic investment in AI at Google, data science and ML engineering teams can now access the AI toolkit used internally to power Google, deploy more useful AI applications, and manage models with confidence.
So far, VertexAI is one of the most powerful AutoML platforms. Besides this, other products by Google Cloud include AutoML Video Intelligence, AutoML Natural Language, AutoML Translation, and AutoML Tables.
Pioneering AutoML
Leaving no stones unturned, Google researchers are continually looking to innovate its AutoML platforms. Earlier this year, Google introduced a new way of programming AutoML based on symbolic programming called PyGlove, primarily used for implementing the symbolic formulation of AutoML.
Here is some of the key research work done by Google over the years, advancing AutoML in highly diverse areas like time-series analysis, computer vision, etc.
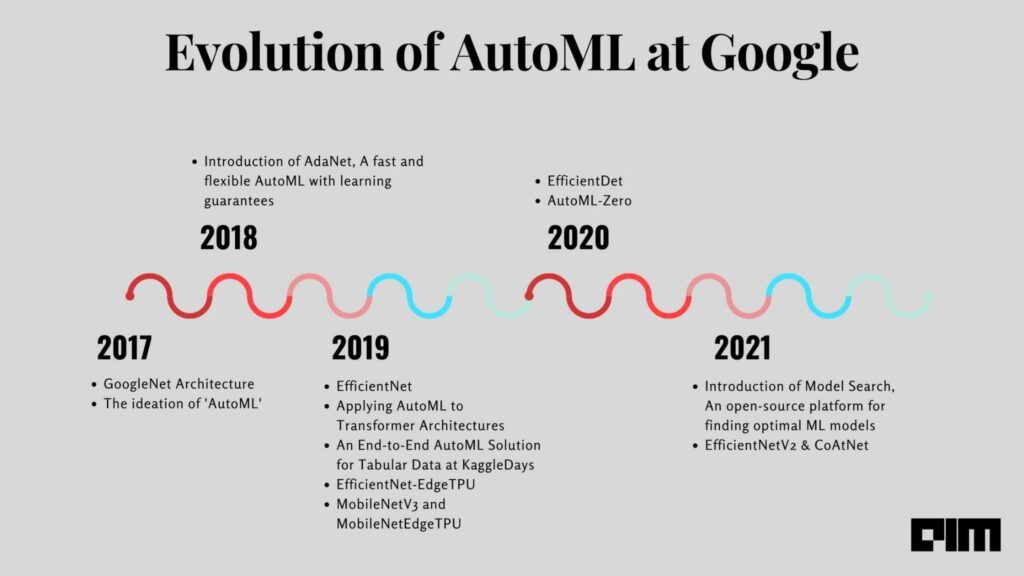
The Winner is
Since 2014, AutoML has been an active area of research and the launch of commercial solutions by big tech, startups and open-source solution providers. Some of the prominent players include Google, DataRobot, Microsoft, H2O.ai, and Amazon. But, the question is, who is leading the AutoML race?
A closer look at the AutoML landscape revealed that Google is upping the game with 162 resources around AutoML on its website and about 153 open-source AutoML APIs, libraries and source code on GitHub. Second in line is H2O.ai, followed by Amazon, DataRobot and Microsoft.
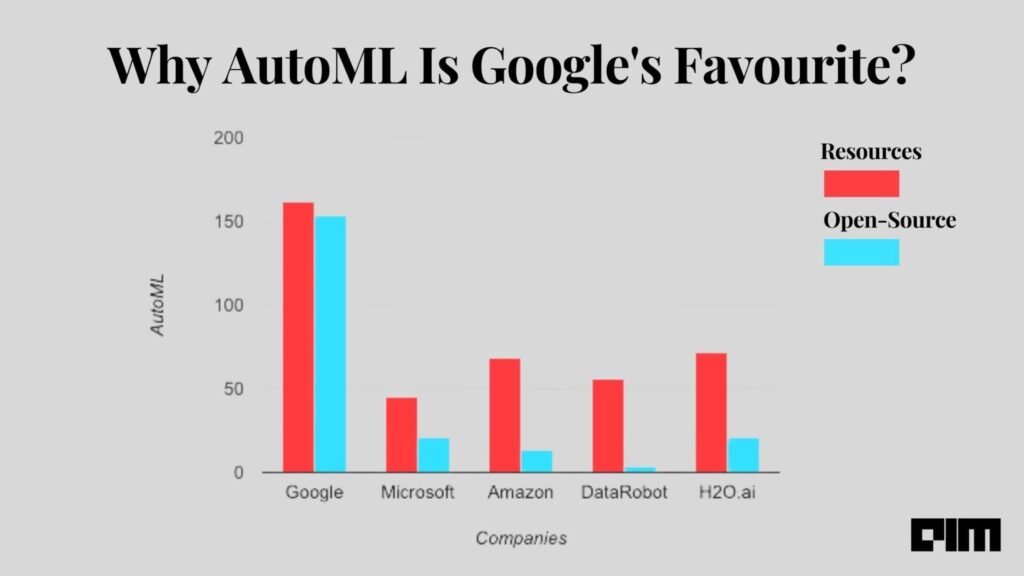
AutoML seems to be Google’s all-time favourite/niche, besides other active areas of research like transformer, federated learning, self-supervised learning, etc.
While Google continues to innovate in the backdrop, the use case of its AutoML platforms is plenty. Companies across the globe are leveraging Google’s AutoML solutions to maintain and deploy AI/ML models. For instance, Imagia’s researchers are developing solutions that use Vertex AI and Cloud AutoML to train multiple AI models and effortlessly run large ensemble learning methods to discover markers for conditions such as chronic and age-related degenerative diseases for preventative assessment.
Similarly, Meredith Digital uses Cloud AutoML Natural Language to train its custom ML models to predict text categories independently without requiring in-house ML expertise.
The future is AutoML
Given the increasing interest and discussion around AutoML coupled with early market adoptions, AutoML shows the enormous potential of changing the machine learning landscape. However, with all the buzz created around AutoML, Google seems to be a clear winner. Hopefully, we will see tough competition in the coming years – in terms of companies releasing more and more open-source and commercial tools to manage and deploy machine learning models (both on-premises and cloud).
According to Research And Markets, the AutoML market generated a revenue of $300K billion in 2019 and is expected to increase to $14.5 billion by 2030. The growth will be fueled by increasing demand for more efficient fraud detection solutions, the growing need for personalised product recommendations, and the rising importance of predictive lead scoring.
Source: https://analyticsindiamag.com