©2021 Reporters Post24. All Rights Reserved.
The Korea Advanced Institute of Science and Technology (KAIST) has announced the development of “RaiBo,” a robodog that can walk robustly even in deformable terrain like sandy beaches.
The research team led by Professor Jemin Hwangbo of the Department of Mechanical Engineering created the quadrupedal robot control technology, according to a press release published by the institute on Thursday.
“The proposed controller can be used without prior information on the terrain, so it can be applied to various robot walking studies,” said Suyoung Choi, the first author of the study.
“It has been shown that providing a learning-based controller with a close contact experience with real deforming ground is essential for application to deforming terrain.”
The team created a system to imitate quadrupedal robot, the force experienced by a walking robot on terrain made of granular materials like sand.
They also developed a structure for an artificial neural network that can walk and make in-the-moment decisions while adjusting to different types of terrain without prior knowledge.
The purpose of this technology’s application to reinforcement learning was to broaden the range of uses for quadrupedal walking robots.
Reinforcement learning is an AI learning technique used to build a computer that gathers information on the outcomes of various actions in a random circumstance and uses that information to carry out a task.
AI-powered robodog – ‘RaiBo’
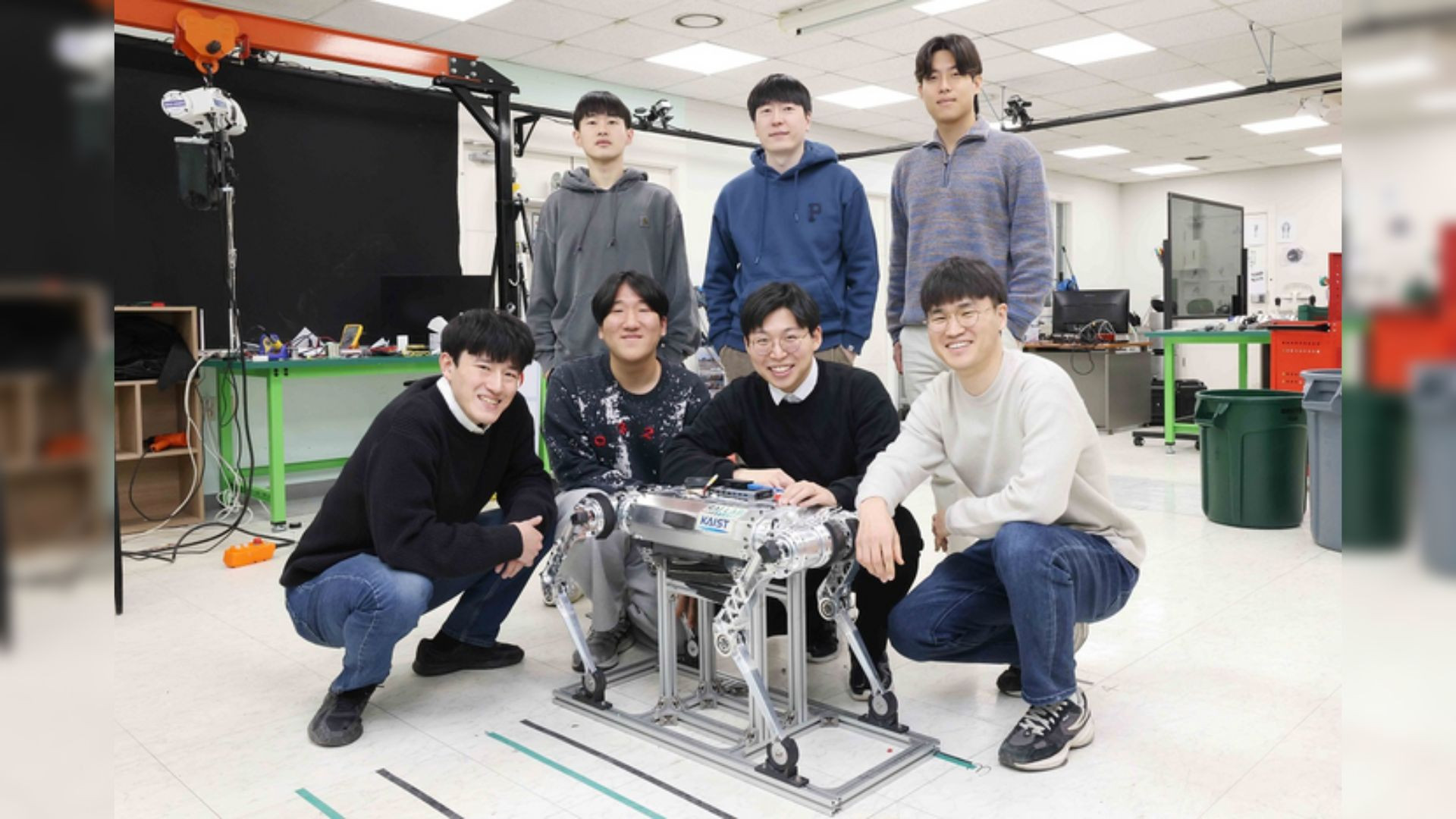
The trained neural network demonstrated its capacity to operate in a variety of environments, including the ability to move quickly and to walk and turn on soft surfaces like an air mattress or sand without losing balance.
Due to the enormous amount of data needed for reinforcement learning, it is common practice to gather data through simulations that closely resemble physical processes in the actual world.
The study team also developed an artificial neural network structure that uses a recurrent neural network to assess time-series data from the robot’s sensors to implicitly predict ground properties.
The learnt controller was installed on the robot “RaiBo,” to demonstrate fast walking at speeds of up to 3.03 meters per second on a sandy beach with its feet entirely buried in the sand.
Without any further programming or revisions to the controlling algorithm, “RaiBo” was able to operate steadily even when used on rougher surfaces like grassy fields and a running track.
The study team’s simulation and learning methodology is anticipated to aid in the development of robots that can carry out practical activities by enhancing the variety of terrains that different walking robots can traverse.
The study was first published in Science Robotics.
Study abstract:
Simulation-based reinforcement learning approaches are leading the next innovations in legged robot control. However, the resulting control policies are still not applicable on soft and deformable terrains, especially at high speed. The primary reason is that reinforcement learning approaches, in general, are not effective beyond the data distribution: The agent cannot perform well in environments that it has not experienced. To this end, we introduce a versatile and computationally efficient granular media model for reinforcement learning. Our model can be parameterized to represent diverse types of terrain from very soft beach sand to hard asphalt. In addition, we introduce an adaptive control architecture that can implicitly identify the terrain properties as the robot feels the terrain. The identified parameters are then used to boost the locomotion performance of the legged robot. We applied our techniques to the Raibo robot, a dynamic quadrupedal robot developed in-house. The trained networks demonstrated high-speed locomotion capabilities on deformable terrains: The robot was able to run on soft beach sand at 3.03 meters per second although the feet were completely buried in the sand during the stance phase. We also demonstrate its ability to generalize to different terrains by presenting running experiments on vinyl tile flooring, athletic track, grass, and a soft air mattress.